Publication at HICSS 2021: Hirt et al. on Sequential Transfer Learning in Networks
January 5, 2021
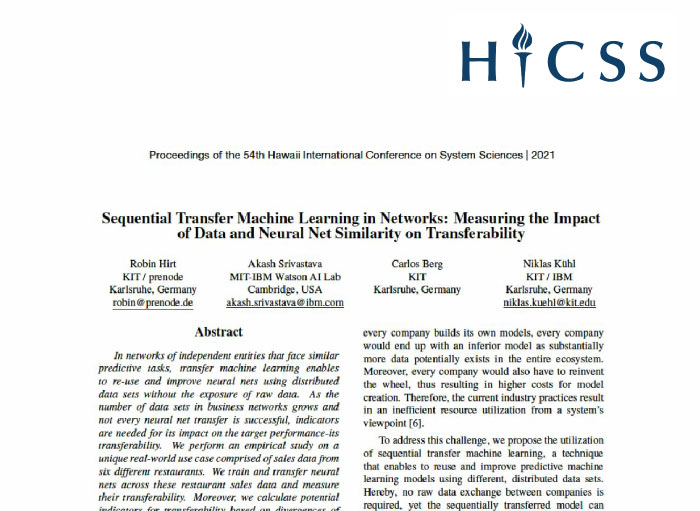
Measuring Impact Factors for ML Model Re-Use
In the recently published Proceedings of the 54th Hawaii International Conference on System Sciences (HICSS), prenode’s co-CEO Robin Hirt explored the possibilities of reusing neural nets on distributed data sets. In this domain there is the issue is that the re-use of machine learning models has a large potential but not every transfer is successful. Therefore, indicators can help to estimate the transferability of neural nets.
In collaboration with the co-authors Akash Srivastava, Carlos Berg, and Niklas Kühl, the team performed an empirical study on a use case involving different restaurants’ sales data. Neural networks are trained across restaurants’ data sets and exchanged with transfer machine learning. The results suggest that indicators like net similarity can be used to estimate model performance.
Transfer Learning Improves ML Performance
The models’ scores show that transfer learning can lead to a significant improvement in the performance of a machine learning system. Therefore, the training of individual models on several, distributed data sets and the following model transfer is a useful way to enhance a system’s utility.
In addition, the team states that pre-trained models can benefit from the training on another data set, as this “outperforms the model built solely on the original distribution” (Hirt et al., 2021).
prenode’s Decentralized Machine Learning software mlx applies this technology and leverages an approach where training data stays on the edge. Therefore, only the individual, locally trained models are exchanged to build a combined centralized model. Learn more about our AI solution here.
Further information on the research is in the paper at hawaii.edu.
Video: Microsoft Intelligent Manufacturing Award 2023 | Meet the winners
Share this article
Read more