Intelligent and adaptive data processing on your edge devices
Edge AI Execution
Deploy AI on the edge devices to realize i.e. predictive maintenance, anomaly detection or process control.
Adaptive AI
Adapt and fine-tune your AI models on scale to account for individual local circumstances and requirements.
Decentralized Learning
Optimize your AI across facilities without exchanging raw data by utilizing federated learning
Why Edge ai?
Build and manage Machine Learning Models in a scalable way on distributed systems
Local, Decentralized, and Fast
Implement real-time data processing directly on the individual machine. This keeps your data within the company. React to changes in milliseconds.
Keeping your company activities running smoothly
ML-based features and services can continue to operate seamlessly even when machines or services are offline. By deploying ML models directly on the edge, our solution ensures that your operations remain unaffected.
Ensuring complete data control
You decide which data you process locally and which you can optionally transfer to a cloud for further processing.
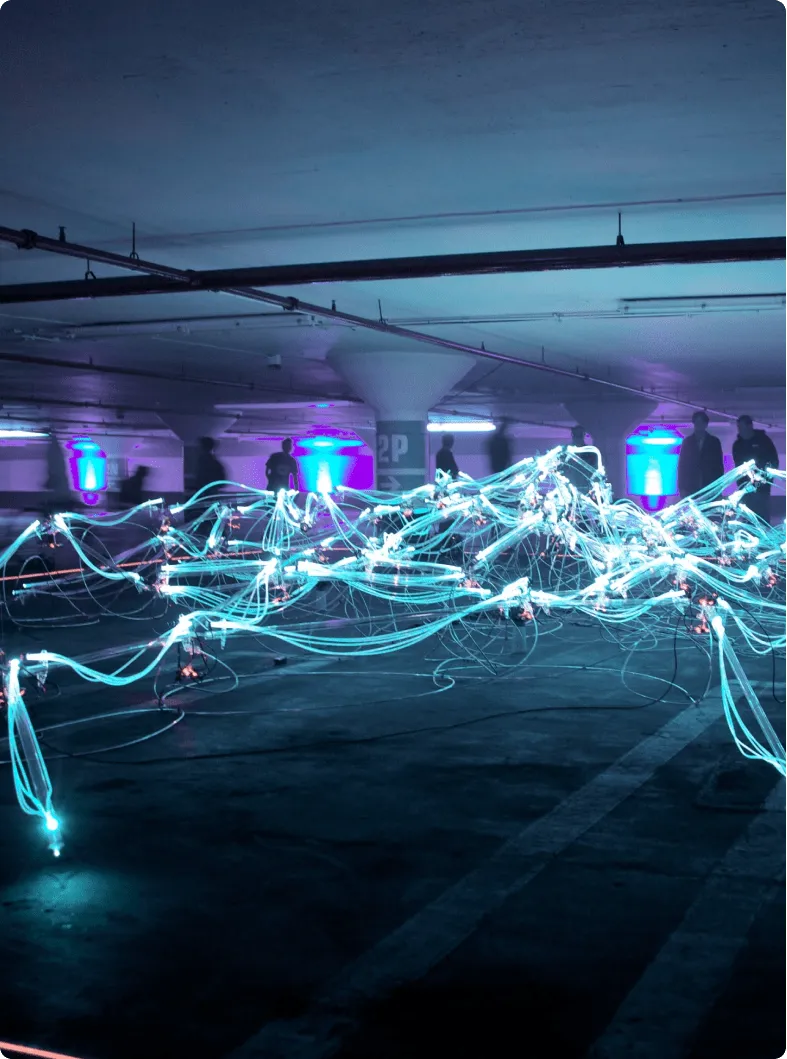
Organizations that want to share data, but are concerned about privacy, should explore a federated learning approach. [...] There is a small yet growing list of vendors using various approaches in that space, including [...] prenode
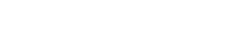
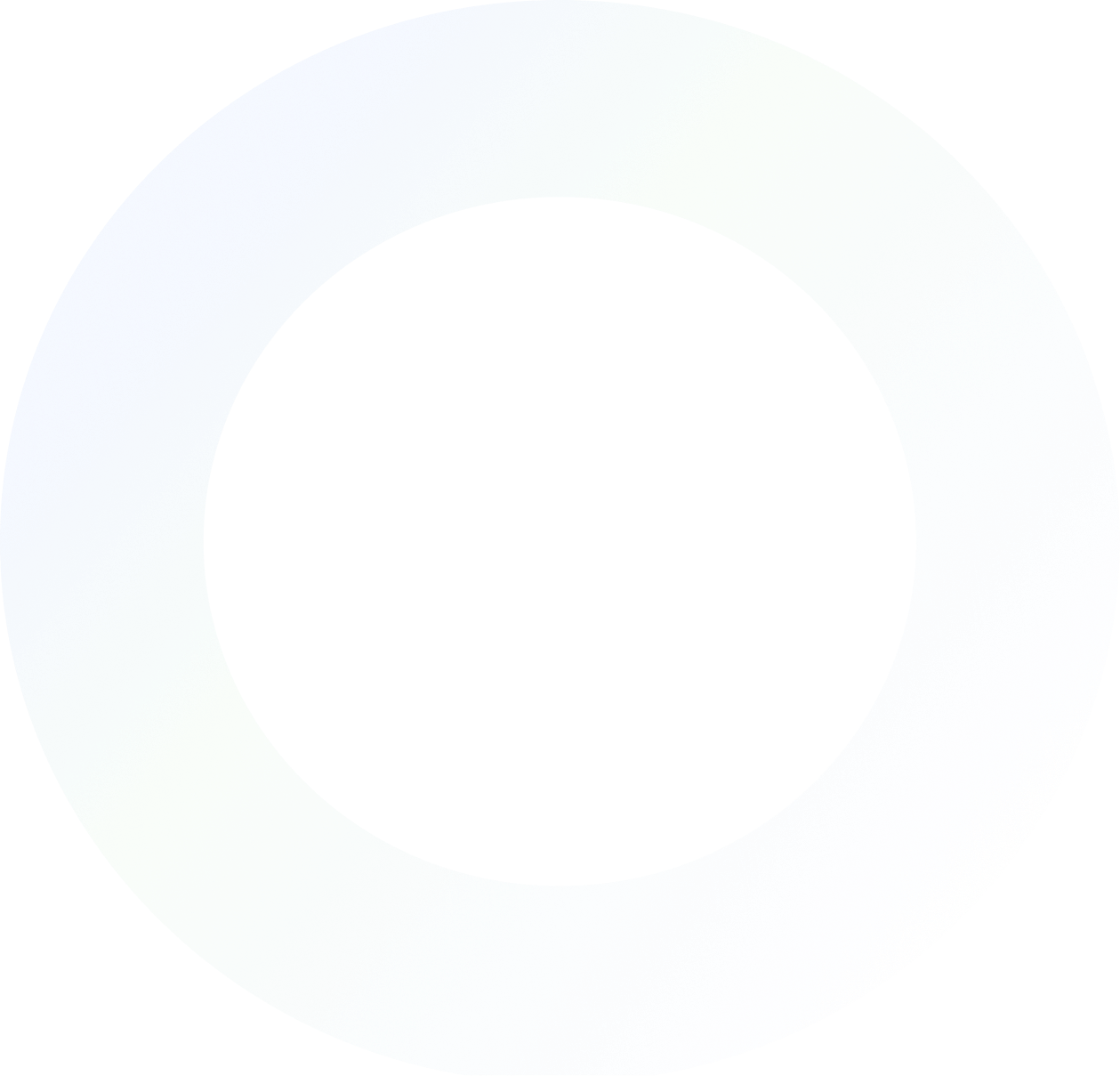

features
Features of our Edge AI solution
Real-time processing of data on the edge
Our Edge AI Solution empowers your devices to analyze data instantly, enabling immediate decision-making without delays.
Reduced reliance on cloud services
Data is processed directly on your devices without the need for constant cloud connectivity, giving the ability to operate in offline or low-connectivity environments and enhancing security.
Improved efficiency and resuced costs
By leveraging Edge AI, your business achieves better performance and accuracy while minimizing costs for data processing, data transfer, infrastructure, and energy.
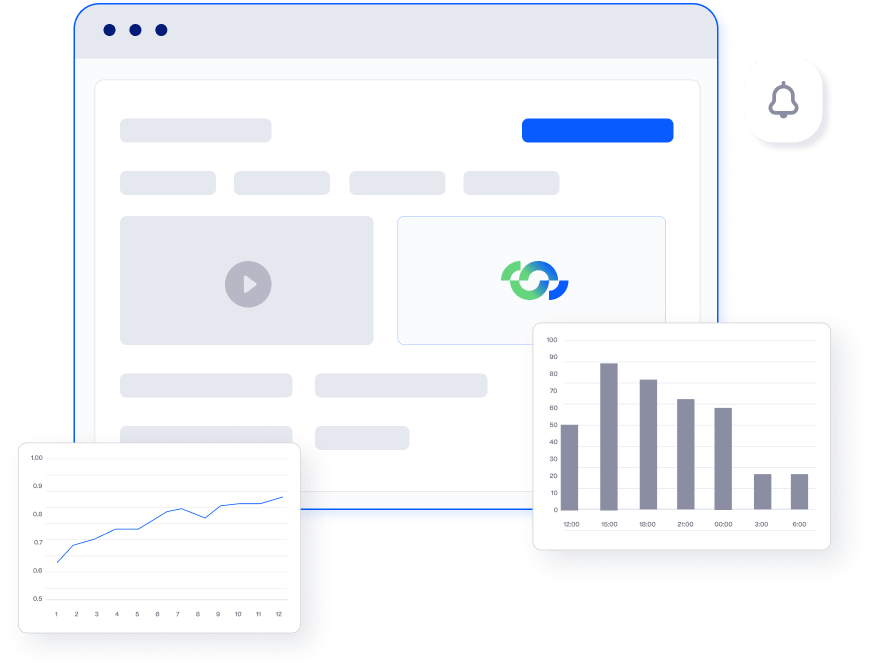

Hardware-agnosticEdge AI Software
Experience seamless integration, flexibility, and compatibility across diverse hardware platforms, ensuring easy deployment and operation on a wide range of devices.
Fine-turning AI models locally
By adaptively fine-tuning your AI models directly on edge devices, you improve accuracy using local data tailored to individual circumstances and requirements.
Federated Learning
Optimize your AI across facilities and devices without exchanging raw data, enhancing security and privacy.
Fueled by the latest technology
Use cases with Adaptive Edge AI
Discover how Industrial Edge AI is changing the manufacturing industry
Vision-based Process Control
Utilizing AI and computer vision technologies to monitor and optimize industrial processes based on real-time visual data analysis.
Condition Monitoring
Applying AI and sensor technologies to continuously monitor the condition and performance of equipment or systems, facilitating predictive maintenance and minimizing downtime.
Anomaly Detection
Identifying and flagging unusual or abnormal patterns in data, enabling early detection of anomalies and potential problems in complex systems.
Operation Parameter recommender
Analyzing data and recommending optimal operating parameters for various processes or systems, optimizing efficiency and production quality while minimizing manual intervention.
Energy Management
Continuously monitoring and analyzing energy consumption patterns to optimize energy usage resulting in cost reductions and improved sustainability.
Consumables Forecast
Leveraging decentralized AI to predict and forecast the usage and availability of consumable resources to optimize supply chain management and production planning.
Case studies
We guide you on the path to Industry 4.0 based on your individual needs
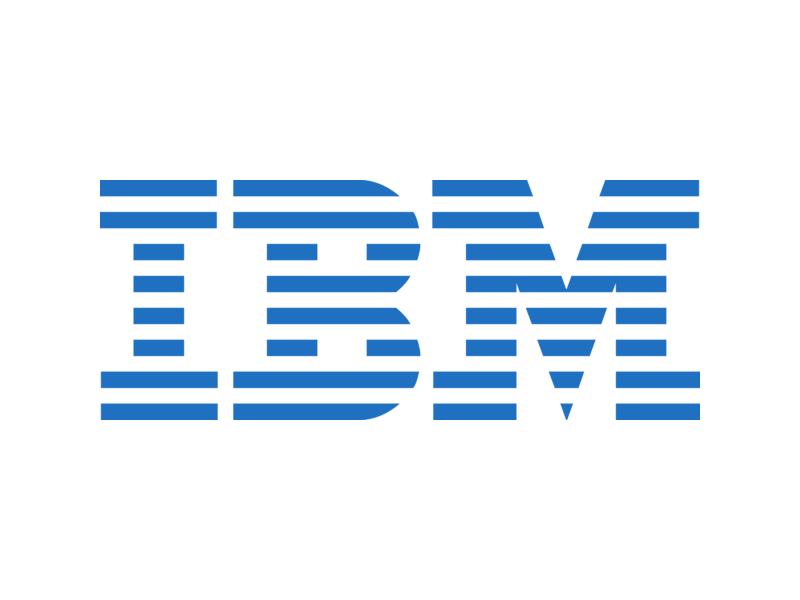
A Smarter Way to Monitor Your Equipment with IBM's Asset Monitoring and prenode Edge AI
Challenge
Many software providers, including IBM, want to enrich their products with machine learning (ML) to deliver AI-based digital services that meet the evolving needs of businesses and customers in today's digital landscape.
However, ML needs enormous amounts of data that cannot easily be accessed because customers are unwilling to share their data due to privacy or high data volumes. In addition, companies often face the challenge of data being distributed across silos, making it difficult to use all the data for ML effectively. It causes knowledge of silos and delayed forecasts.
IBM actively pursued a technical solution to overcome data challenges and enhance their IBM Maximo® Monitor portfolio (a remote asset monitoring application) with AI capabilities.
How we helped
To resolve these challenges, our decentralized AI solution, prenode mlx, offers a comprehensive infrastructure for on-device machine learning, enabling the training of models on federated and isolated data sets. This solution allows training ML models without sharing data with a central entity and keeps the data on edge at individual sites to ensure privacy, security, and accurate predictions.
In collaboration with IBM, we developed a Hammer-Rig showcase where mlx seamlessly integrated into IBM's infrastructure, demonstrating its capability to predict overheating events by analyzing data from multiple pumps. Our decentralized AI software analyzed sensor data from each individual rig, accurately predicting overheating events and sending the predictions to IBM Maximo Monitor platform through IBM Cloud. This enables machine operators to quickly receive warnings when an overheating event is predicted.
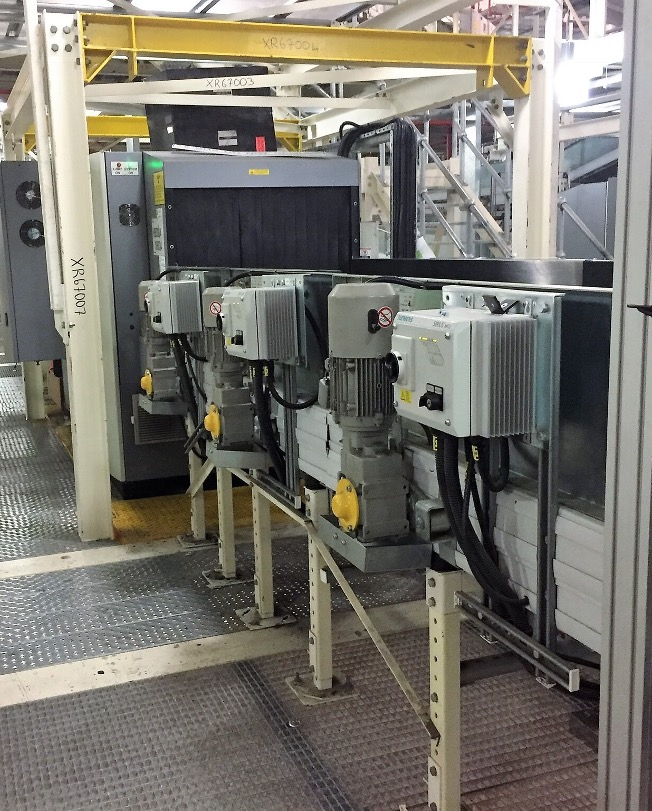
Learn more about Edge AI
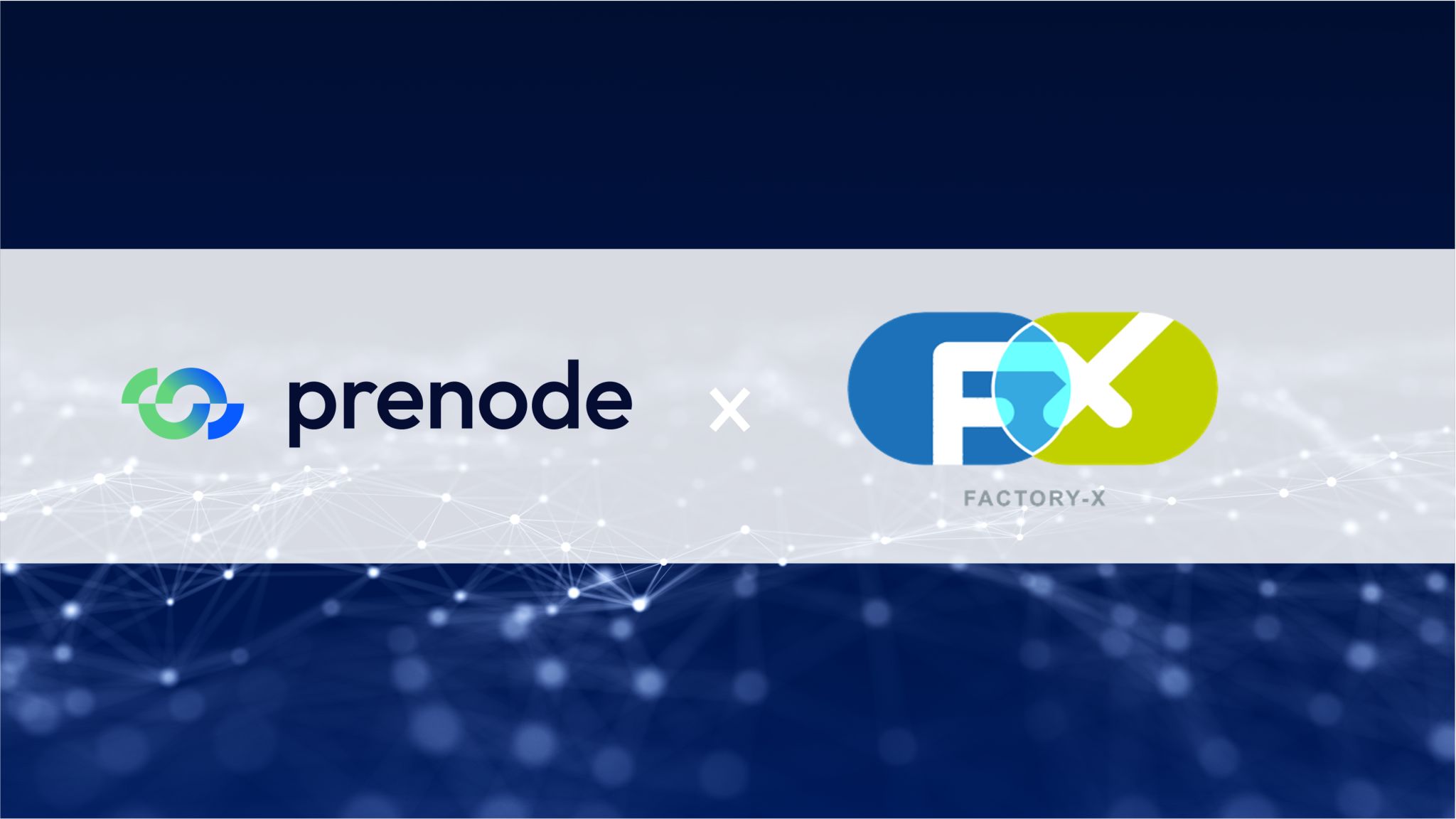
April 18, 2024
Factory-X: The digital ecosystem for manufacturing — and prenode is on board!

August 4, 2023
prenode continues to shine in the German AI Startup Landscape for the third consecutive year

October 1, 2019
StartUpSecure Initiative: Research Project Supporting the National IT-Strategy
Are you looking for a support in building your AI applications?
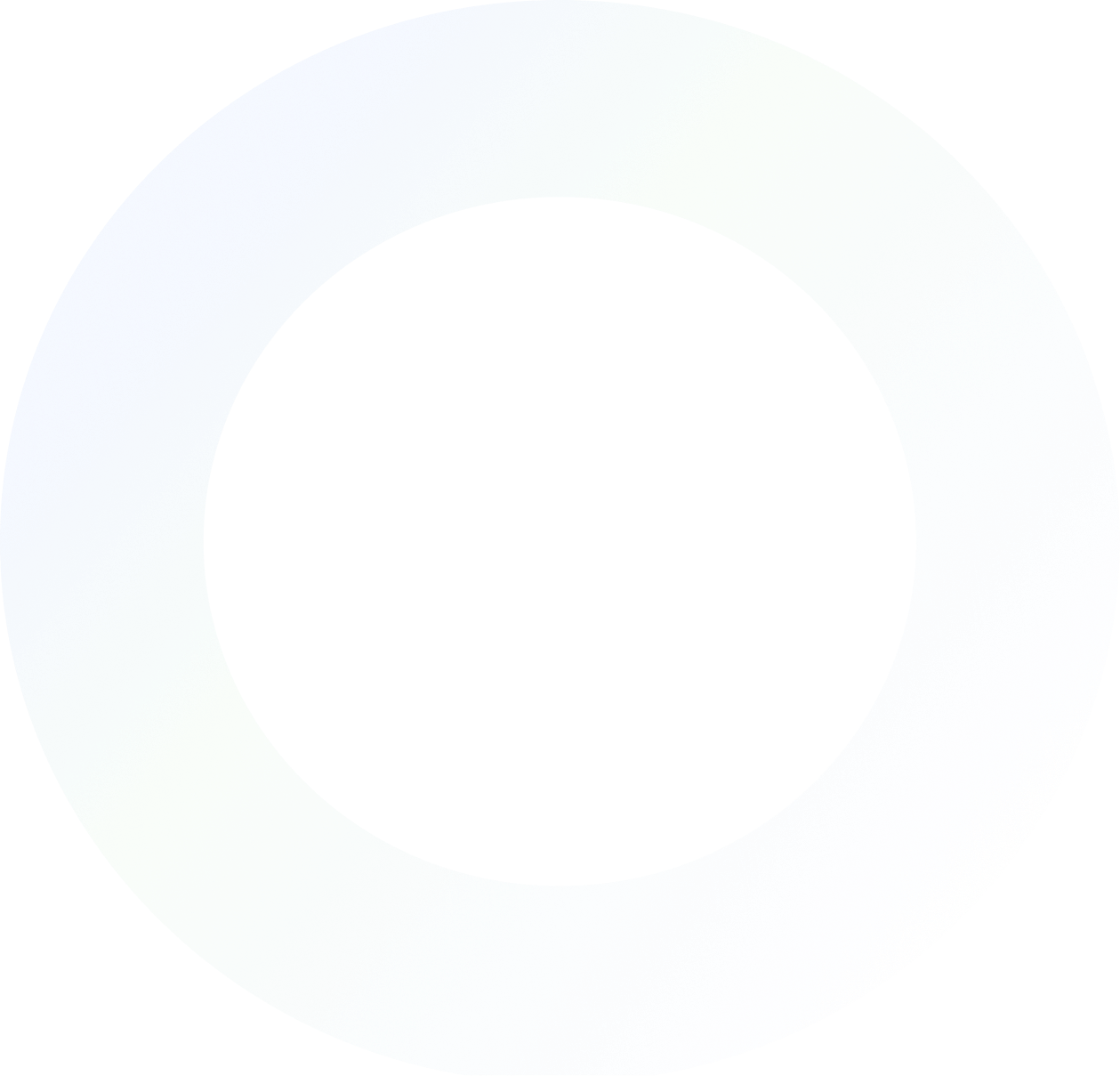