How Edge AI Facilitates Smart Manufacturing
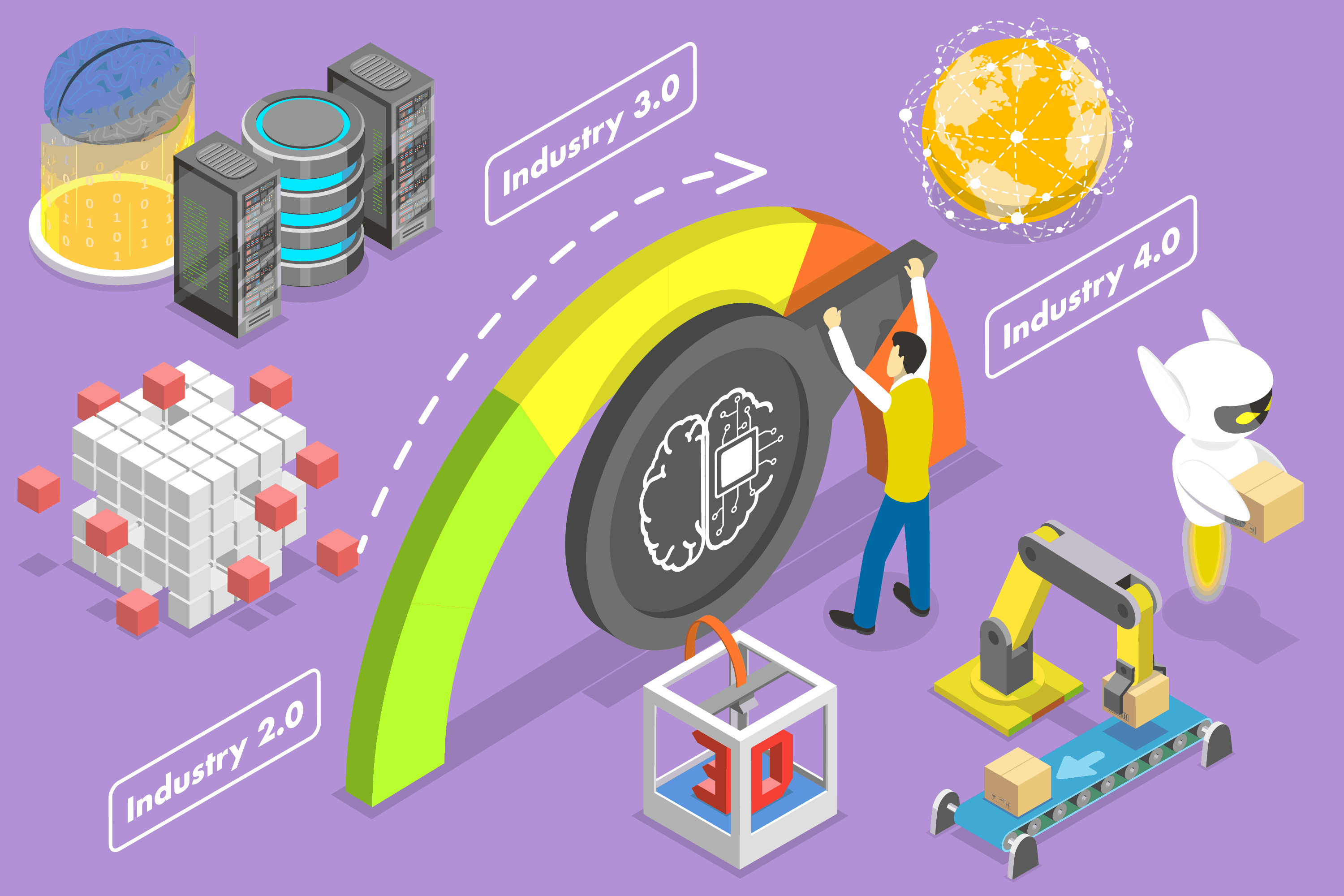
Numerous companies and manufacturers have prioritized digital transformation as a cornerstone of their strategies. Artificial intelligence (AI) has taken center stage in this transformation, becoming an integral part of their production processes. The goal of digital transformation is not just to reduce costs, it is also about increasing operational efficiency, enhancing existing products, and innovating new products and services.
Edge AI benefits from improved edge device computational capabilities, boosting privacy, security, and real-time decision-making efficiency. This article delves into the significance of Edge AI and its impact on manufacturing in the future.
What is Edge AI?
IIn contrast to the traditional setting where inference occurs within a cloud computing network, Edge AI enables the model to operate directly on the edge device without the need for continuous external connectivity. This paradigm allows us to retain sensitive data at the data source and rapidly act on new input without the detour to a cloud environment.
Edge AI involves the deployment of AI algorithms and models on edge devices or within edge computing nodes, such as smartphones, sensors, or edge servers. The primary goal of Edge AI is to process data locally on these devices, close to the data source, rather than relying solely on centralized cloud-based AI systems.
Here is how Edge AI typically works:
- Data Collection: Edge AI begins with the collection of data from various sensors, devices, or sources at the edge of the network. These data sources can include cameras, sensors, microphones, and more, depending on the specific application.
- Data Preprocessing: The collected data is preprocessed on the edge device to clean, filter, and format it for analysis. Preprocessing may involve tasks such as noise reduction, data compression, or data normalization to ensure the data is suitable for AI processing.
- Inference: Inference is the core of Edge AI. Machine learning models, which have been trained on larger datasets, are deployed to the edge device. These models can be deep neural networks or other AI algorithms. When new data arrives at the edge device, the deployed model processes this data to make predictions, classifications, and makes decisions in real-time. This inference happens locally on the edge device, avoiding the need to send data to a remote server for processing.
- Decision-Making: The results of the inference are used to make immediate decisions or trigger actions. For example, in autonomous vehicles, Edge AI can recognize obstacles in real-time and steer the vehicle to avoid collisions. In manufacturing, Edge AI can identify defects on the production line and take corrective actions or assess the criticality of a situation to assist a human (remote) operator.
- Feedback Loop: In some cases, the results of Edge AI processing can be sent back to a central server for further analysis, model updates, or long-term storage. This feedback loop helps improve the performance of Edge AI models over time by incorporating new data and insights.
Why Use Edge AI in Manufacturing?
By processing data locally and reducing reliance on cloud services, Edge AI ensures consistent performance in environments with limited network connectivity, while its adaptability and cost-efficiency make it a valuable tool for streamlining manufacturing processes. There are several advantages of implementing Edge AI in manufacturing:
- Real-time Decision-Making
Production lines require instantaneous responses to changing conditions. Edge AI allows for real-time data analysis and decision-making, ensuring that critical adjustments can be made instantly.
- Keeping Data Secure at the Edge
Edge AI can help maintain data privacy by processing sensitive information locally, without transmitting it to the cloud. This significantly lowers the risk of data breaches and ensures that critical data remains firmly under the user's control.
- Improved Efficiency and Reduced Costs
By embracing Edge AI, your business can achieve enhanced performance and accuracy while minimizing expenses related to data processing, data transfer, infrastructure, and energy consumption.
- Downtime Minimization
Edge AI's ability to predict equipment failures and enable proactive maintenance minimizes production downtime.
- Customization and Adaptability
Edge AI solutions can be tailored to meet specific application requirements, and they can be easily updated and adapted to evolving needs, providing a high degree of flexibility and responsiveness to changing demands.
What Are the Common Use Cases of Edge AI in Industry?
Edge AI is particularly useful in applications that require rapid response, data privacy, security, or in remote locations with limited network connectivity. It can be used in various sectors, such as industrial machinery, manufacturing, autonomous vehicles, real-time healthcare monitoring devices, and beyond. Within industrial manufacturing, prevalent applications include:
- Smart (remote) Operation: Edge AI technology can help remote machine operators by giving them information about the machine's status and alerting them when something unusual happens. It can use live machine data and camera feeds, so operators can solve problems quickly. This reduces the need for on-site workers, helping with labor shortages.
- Predictive Maintenance: Edge AI can analyze sensor data to predict equipment failures in real-time, allowing maintenance teams to respond promptly to predictions, mitigate potential damages, and enhance maintenance practices.
- Quality Control and Inspection: Defect detection is an essential part of the manufacturing process. Devices that use Edge AI-powered computer vision systems inspect products and components for defects. This technology can identify even subtle defects, reducing waste ensuring consistent product quality, and improving manufacturing efficiency.
- Energy Management: Energy costs impact manufacturing expenses and sustainability. Edge AI can monitor and optimize energy consumption, can lead to cost savings, and thereby reduce the carbon footprint.
- Real-time Process Optimization: Edge AI can analyzes data from various sensors, such as temperature, pressure, and humidity sensors, to optimize manufacturing processes in real-time. It can adjust machine settings, resource allocation, and production parameters to maximize efficiency.
- Worker Safety: Worker safety is a priority in manufacturing. Edge AI systems can monitor worker movements and conditions to provide real-time alerts, preventing accidents and ensuring compliance.
Federated Learning in Edge AI
Federated learning, a machine learning approach, involves acquiring knowledge from distributed data sources. It enhances Edge AI by using a model iteration technique instead of directly accessing data from devices, effectively addressing privacy concerns while facilitating learning across diverse edge systems.
Summary
Industry 4.0 evolution depends on the transition to Edge AI, particularly following the era of cloud computing and the IoT. The adoption of Edge AI becomes the logical next step, as it holds the key to unlocking Industry 4.0's full potential.
At prenode, we are dedicated to steering and supporting enterprises on their path to intelligent manufacturing, navigating each step of the process. This entails evaluating the existing situation, conducting a comprehensive analysis, formulating a strategic roadmap, and executing the integration of Edge AI technology. Utilizing the approach of Federated Learning, we fine-tune AI systems across multiple facilities without the need for raw data exchange to ensure your data privacy.
Share this article